While global statistics on the number of companies using artificial intelligence (AI) for sustainability are still emerging and vary depending on the definition and scope, several reports and surveys offer valuable insights. A significant majority of C-suite executives recognize the importance of AI for achieving their sustainability goals. An edX survey in September 2024 found that 75% of C-suite respondents believe their company will not be able to achieve its sustainability targets without AI. Together with the research firm Workplace Intelligence, edX surveyed 1,800 global knowledge workers and 600 US C-suite executives to gain unique insight into how AI can be used to accelerate corporate sustainability efforts, which includes environmental, social, economic, cultural, and ethical impacts of a company on its stakeholders. Interestingly, despite the belief among most respondents that AI is essential to reaching sustainability targets, 65% acknowledge they “have no idea how” to operationalize AI to support sustainability.
Key Insights
- Increasing adoption: While the majority see AI as crucial, the actual implementation is still in relatively early stages for many. The edX survey indicated that while 96% of C-suite respondents at companies using AI for sustainability reported improved progress, 65% admitted they “have no idea how” to operationalize AI for this purpose.
- Early implementation: A Salesforce survey in July 2024 revealed that nearly half of sustainability professionals have begun using or experimenting with AI in their sustainability programs, with 20% having fully implemented it and 29% still in the experimentation phase.
- Importance for IT leaders: A 2024 IDC report stated that over 76% of IT decision-makers worldwide consider AI and its derivatives to be “critical” or “very important” for their organization’s sustainable transformation journey, and over 40% say that at least half of their AI spending has a sustainability-related component.
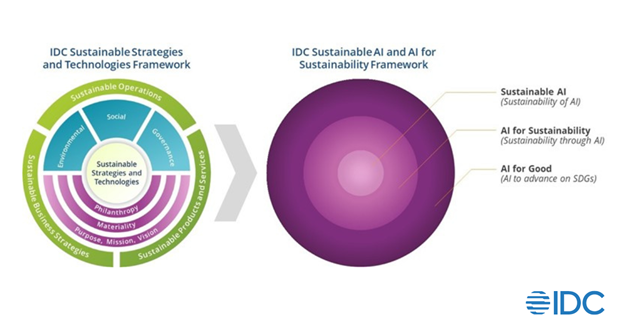
AI is a powerful tool with the potential to revolutionize sustainability efforts that enhance business value and drive meaningful environmental impact. These technologies can be used to:
- Optimize energy: Smart grids, building automation, and renewable energy management powered by AI reduces waste and maximizes efficiency
- Monitor the environment: AI analyzes large datasets from satellites and sensors to track deforestation, wildlife, pollution, and climate change
- Improve agriculture: Precision agriculture uses AI to optimize resource use, reduce waste, and improve yields
- Streamline waste management: AI optimizes collection routes and automates recycling processes
- Enhance transportation: AI enables traffic optimization, efficient route planning, and the development of autonomous vehicles
- Track and reduce carbon footprints: AI monitors emissions and identifies reduction opportunities across industries and for individuals
These technologies offer enhanced efficiency, monitoring, data-driven insights, automation, and accelerated innovation for a more sustainable future. However, challenges like energy consumption of AI, data privacy, algorithmic bias, and the need for transparency must be addressed.
Case Studies
Google’s DeepMind utilizes deep reinforcement learning algorithms to analyze enormous amounts of historical and real-time data from thousands of sensors within their data centers. This data includes temperature, power usage, pump speeds, and other operational parameters. The AI system has learned to predict how different cooling adjustments affect future energy consumption and the overall Power Usage Effectiveness (PUE), which is a key metric for data center efficiency. Initially, the AI provided recommendations to human operators. However, the system evolved to directly control the cooling systems, adjusting parameters like cooling tower speeds, chillers, and fan speeds autonomously while adhering to strict safety constraints. Human operators retained oversight and the ability to intervene if necessary. Google reported that DeepMind’s AI was able to reduce the energy used for cooling by up to 40% in the data centers where it was deployed. By significantly reducing energy consumption for cooling, Google has also lowered the carbon footprint of its data centers, contributing to their broader sustainability goals.
GE’s Digital Wind Farm refers to General Electric’s (now part of GE Vernova) initiative that integrates digital technologies with wind turbines and wind farm operations to enhance efficiency, reduce costs, and increase energy production. It is not a physical wind farm in a specific location, but rather a comprehensive hardware and software solution. The Digital Wind Farm merges GE’s wind turbine technology with a digital infrastructure powered by software platforms like Predix (GE’s industrial internet platform).
These turbines are equipped with sensors and controls that allow them to adapt to changing wind conditions and communicate with the digital infrastructure. For each physical turbine, a virtual copy or “digital twin” is created in the cloud allowing for simulations, performance monitoring, and predictive analysis. The digital twin reacts to real-world conditions and calculates potential adjustments.
Amazon uses its Packaging Decision Engine, an AI model, to determine the most efficient packaging options, which has helped eliminate over 2 million tons of packaging material since 2015. The company also uses AI to prevent food waste by monitoring produce for imperfections and to reduce returns by helping customers find the perfect fit for fashion items. The AI model provides:
- AI-powered optimization: This AI model uses a combination of deep learning, natural language processing (NLP), and computer vision to determine the most efficient packaging for each product shipped to customers.
- Data-driven decisions: The engine analyzes various data points including product characteristics, product descriptions, historical data, and customer feedback.
- Predictive capabilities: The model predicts whether a product needs protective packaging or if it can be shipped in its original manufacturer’s packaging (through Amazon’s “Ships in Product Packaging” program). It can also determine if a fragile item requires a sturdier box versus a mailer.
- Continuous learning: The Packaging Decision Engine is constantly learning and refining its decisions as it processes new data about products, packaging options, and customer feedback.
These case studies highlight the diverse applications of AI and machine learning (ML) in driving sustainability across various industries, leading to increased efficiency, reduced waste, better resource management, and a smaller environmental footprint.
Conclusion
As highlighted by the success of Google’s DeepMind, GE Vernova’s Digital Wind Farm, and Amazon’s Packaging Decision Engine, AI and ML offer companies the ability to optimize resource utilization, minimize waste, and significantly reduce environmental impact across diverse operations. These technologies provide enhanced efficiency, intelligent monitoring, data-driven insights, and automation crucial for navigating the complexities of building truly sustainable supply chains and business practices.
Companies should begin by identifying key areas where data-driven insights and automation can yield the greatest environmental and economic benefits. They should embrace pilot projects, learn from the outcomes, and scale successful initiatives. If your organization is looking for insights and strategies for how best to utilize AI capabilities for sustainability initiatives, please contact Canopy Edge for an initial consultation.